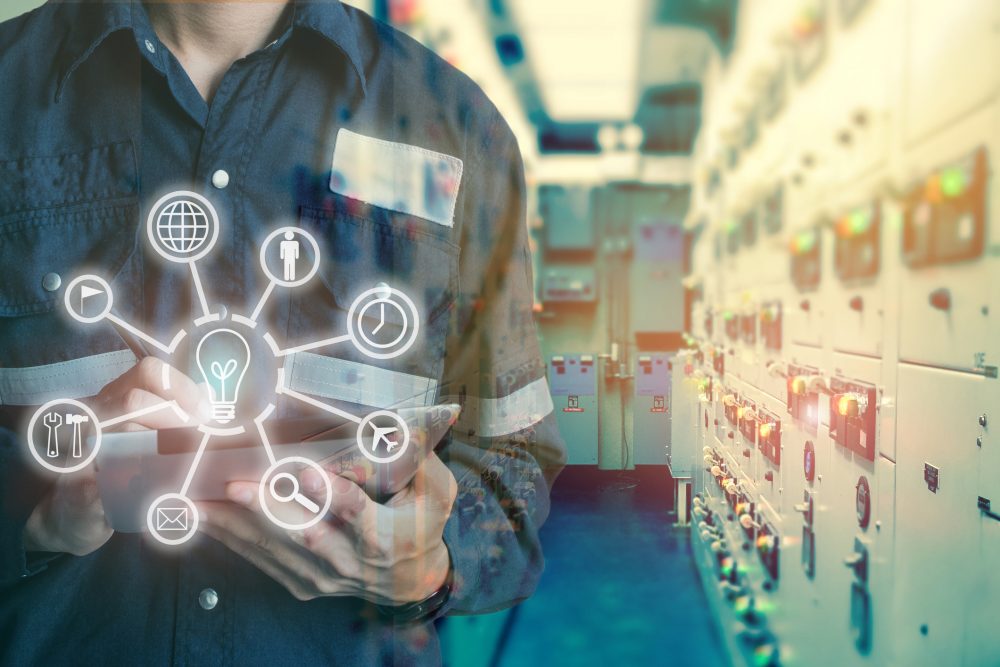
Maintenance 4.0: Predictive Maintenance
Industrial maintenance in the fourth wave on the test bench: Technical failures and reduced production quality due to wear and tear of machines and materials lead to massively increasing costs in industry. The change from reactive to preventive measures is inevitable. In the article, you can read about potentials, challenges and practical examples.
What is Predictive Maintenance?
Predictive maintenance is understood to mean proactive maintenance – for example, in the context of manufacturing processes. Maintenance is based on machine and production data in real time, which provides information about the condition of the equipment, its useful life, performance, and so on. In this way, corrective measures can be planned and implemented before critical situations arise. Impending damage or even complete production downtime can be largely prevented with this method.
What are the advantages?
- With predictive maintenance, even minor deficiencies or quality drops in production become quickly visible and can be rectified immediately. Unplanned downtimes or even machine failures due to defects and damage to equipment can thus be largely avoided. This saves costs in the long term and safeguards manufacturing processes.
- Regularly maintained machines have a longer service life. Since the purchase of new plant and equipment is particularly cost-intensive, the long-term use of existing machines has a significantly positive effect on the budget.
- High-quality maintenance and comprehensive service require trained personnel. Predictive maintenance also makes it easier to plan this expenditure of resources. Maintenance resources can therefore be used efficiently, and the ideal time for service work is determined in concrete terms.
- Costs are also saved in spare parts management: regular data collection also increases the ability to plan the need for spare parts and their procurement. Stock levels can be replenished particularly efficiently as a result, which can have an impact on the size of warehouses required.
- The data collected is also useful for another purpose: process optimization. The data sets enable conclusions to be drawn about the efficiency of processes and the performance of plants. Resulting improvements based on data analysis leads to higher productivity.
What are the challenges?
Successful predictive maintenance requires immense amounts of data (Big Data) – in order to be able to make reliable statements and thus provide relevant assessments for planning. They come in a wide variety of forms and units. For example, oscillations or vibrations, sounds and volume levels, temperatures, liquid quantities, fill levels, conductivity, moisture levels or even contamination levels are included. This task is also where most of the hurdles of predictive maintenance lie:
- The collection and processing of the huge amounts of information is not manageable in practice for many companies. Above all, filtering relevant and irrelevant data is a major challenge.
- Data collection also requires special analysis and monitoring tools. For example, sensitive sensors and sufficient digital interfaces are indispensable. If the machines are not yet available for this or are already somewhat outdated, they have to be retrofitted, which leads to high expenses in the short term. These systems also require regular inspection and maintenance – another cost factor!
And because even the most innovative processes do not function completely autonomously, it is important not to forget the specialist personnel who deal with the processes and tasks involved in predictive maintenance – from the programmer to the electrician on site.
Predictive Maintenance: How it works
A primary component of predictive maintenance is condition monitoring. This involves the continuous collection of large amounts of different data through system monitoring and measurements and subsequent comparison with older values.
AI-assisted mechanisms facilitate the handling of Big Data. Systems are taught by machine learning how certain parameters, factors and environmental influences correlate with each other and which conclusions are to be drawn from them at which points in time. The more data is collected, the more complex and specific the conclusions and correlations that the system throws out become. With each new scenario, new insights emerge from which predictive maintenance can benefit in the future.
Predictive Maintenance: The Applications
Numerous industrial fields have already discovered predictive maintenance for themselves. The use of the methodology is particularly popular in the following areas:
- Wind power technology: Sensors in the engine or landing gear reveal small defects and prevent costly total failures.
- Modern vehicle maintenance & e-mobility: Intelligent, networked cars independently and immediately forward defects and other relevant information about the vehicle’s condition to the manufacturer, which means that model or batch defects can be detected and repaired more quickly. In electric vehicles, the method is used for condition assessment and forecasts about battery condition.
- Aircraft technology: It is obvious that early fault detection is essential in aviation. If aircraft cannot take off due to prolonged maintenance, this causes far-reaching chain reactions for the airlines and enormous costs.
- Rail maintenance: Predictive maintenance can also prevent disruptions and delays in rail operations: Gaps in the track are quickly detected and defective switches are replaced.
- Power supply: In order to be able to guarantee a continuous power supply, early warning signs are particularly important for utility service providers. Intelligent systems detect differences between supply and demand in real time and enable rapid action within the industry.
- Oil and gas industry: When oil and gas refining equipment is located in deepwater locations, its condition is difficult to estimate using traditional maintenance approaches. Predictive maintenance enables consistent quality standards and increased service life of the machines used.
- Diverse production operations: Even in the context of classic manufacturing and production processes, delayed repairs often cause major damage to the production process and the quality of the products. High costs and economic losses are the result.
Maintenance 4.0: Maintenance instead of servicing!
Predictive maintenance has already achieved a high level of importance in various industries – but the potential has not yet been fully exploited. Dealing with big data is clearly the biggest challenge. However, the developments and forecasts are promising, and the basic tenor is: maintenance, not servicing!
Predictive maintenance will become more and more prevalent in the future. However, the prerequisite for successful use and establishment is and remains that both the technical and human resources for collecting and processing the immense amounts of data are available. It is therefore advisable to approach the matter slowly instead of trying to convert the entire maintenance area in one go. This prevents surprises if the time, personnel or cost estimates do not quite match reality. But one thing is clear: in the long term, there is no way around predictive maintenance.
Would you like to learn more about the topic of Industry 4.0? Then click through our Industry 4.0 category, where we get to the bottom of traceability in production processes, for example, or look at the use of RFID components. Read online now and find out what’s new!